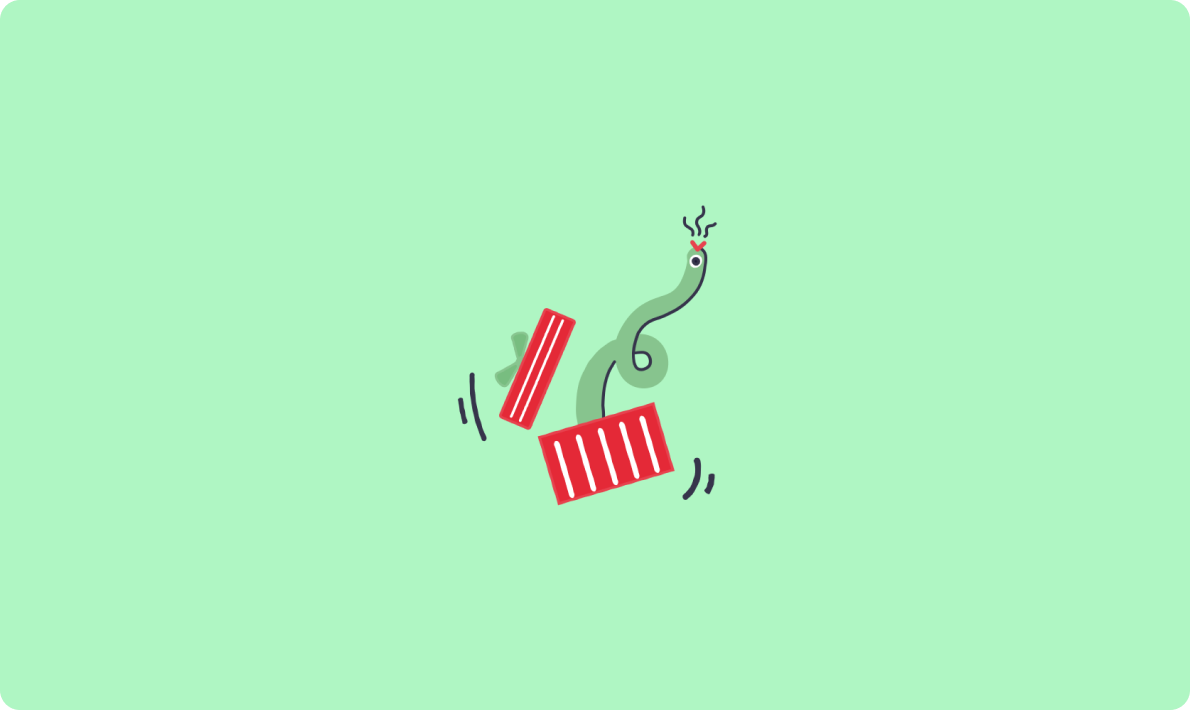
API網(wǎng)關(guān)如何發(fā)展:更輕、更智能、云原生
? ? ? ?當(dāng)解決具體問題時(shí),這些模塊與LLM進(jìn)行多輪對(duì)話。這是基于LLM的自治代理的典型情況,其中動(dòng)態(tài)創(chuàng)建鏈并按順序執(zhí)行,同時(shí)多次輪詢LLM。
? ? ? ?下圖是LangSmith[1]的界面,從圖中可以看到使用的tokens總數(shù)以及兩個(gè)延遲類別。
? ? ? ?此圖顯示了Trace部分,其中包含為該代理創(chuàng)建的完整鏈,以及輸入和輸出。LangSmith在鏈的每一步都給出了詳細(xì)的分解,包括成本(tokens)和延遲。
會(huì)話和狀態(tài)歷史記錄(上下文)存儲(chǔ)在內(nèi)存模塊中,這使代理可以參考思維過程的先前部分,并可能從歷史記憶采取不同的路線。
為了驗(yàn)證ToT技術(shù)的有效性,本文實(shí)現(xiàn)了一個(gè)基于ToT的代理來解決數(shù)獨(dú)難題。
論文[2]實(shí)驗(yàn)結(jié)果表明,ToT框架可以顯著提高數(shù)獨(dú)解謎的成功率
? ? ? ?論文指出的一個(gè)漏洞是LLM是基于前面的序列生成內(nèi)容,而忽略了向后編輯。然而,當(dāng)我們?nèi)祟惤鉀Q一個(gè)問題時(shí),如果派生的步驟不正確,我們很可能會(huì)回溯到以前的迭代。這種回溯方法否定了LLM達(dá)到不確定或無答案場(chǎng)景的危險(xiǎn)。
? ? ? ?其次,為了建立確保正確性,我們?nèi)祟惖囊环N做法是在解決問題的每一步都進(jìn)行測(cè)試,這確保了最終解決方案的可信度。本文統(tǒng)計(jì)了自回歸語言模型在基于以前的token生成新token時(shí),不會(huì)顯式執(zhí)行邏輯正確性檢查,這限制了LLM糾正自身錯(cuò)誤的能力。隨著模型生成更多的tokens,一個(gè)小錯(cuò)誤可能會(huì)被放大,這通常被稱為級(jí)聯(lián)。因此這會(huì)導(dǎo)致生成質(zhì)量下降,并使其難以從錯(cuò)誤中恢復(fù)。級(jí)聯(lián)很早就被認(rèn)為是手動(dòng)創(chuàng)建提示鏈的一種危險(xiǎn)。然而,考慮到自主代理在運(yùn)行中創(chuàng)建了一系列提示,它仍然容易受到級(jí)聯(lián)的影響。
該策略[2]通過LLM和提示器代理之間的多輪對(duì)話來解決問題。
上圖顯示了四種方法的成功率:zero-shot(zs)、one-shot(os)、few-shot(fs)和Tree-of-Thought(tot)。
? ? ? ?以下是ToT代理的完整代碼,您可以將其復(fù)制并粘貼到筆記本中。您需要更新的只是OpenAI API密鑰和LangSmith API密鑰。
pip install langchainpip install langchain_experimentalpip install -U langsmithpip install openai
#######
import osfrom uuid import uuid4
unique_id = uuid4().hex[0:8]os.environ["LANGCHAIN_TRACING_V2"] = "true"os.environ["LANGCHAIN_PROJECT"] = f"Agent Tot"os.environ["LANGCHAIN_ENDPOINT"] = "https://api.smith.langchain.com"os.environ["LANGCHAIN_API_KEY"] = "xxxxxxxxxxxxxxxxxxxxxxxx"os.environ['OPENAI_API_KEY'] = str("xxxxxxxxxxxxxxxxxxxxxxxx")
#######
from langchain.llms import OpenAIllm = OpenAI(temperature=1, max_tokens=512, model="text-davinci-003")
#######
sudoku_puzzle = "3,*,*,2|1,*,3,*|*,1,*,3|4,*,*,1"sudoku_solution = "3,4,1,2|1,2,3,4|2,1,4,3|4,3,2,1"problem_description = f"""{sudoku_puzzle}
- This is a 4x4 Sudoku puzzle.- The * represents a cell to be filled.- The | character separates rows.- At each step, replace one or more * with digits 1-4.- There must be no duplicate digits in any row, column or 2x2 subgrid.- Keep the known digits from previous valid thoughts in place.- Each thought can be a partial or the final solution.""".strip()print(problem_description)
######## The following code implement a simple rule based checker for # a specific 4x4 sudoku puzzle.#######
from typing import Tuplefrom langchain_experimental.tot.checker import ToTCheckerfrom langchain_experimental.tot.thought import ThoughtValidityimport re
class MyChecker(ToTChecker): def evaluate(self, problem_description: str, thoughts: Tuple[str, ...] = ()) -> ThoughtValidity: last_thought = thoughts[-1] clean_solution = last_thought.replace(" ", "").replace('"', "") regex_solution = clean_solution.replace("*", ".").replace("|", "\\|") if sudoku_solution in clean_solution: return ThoughtValidity.VALID_FINAL elif re.search(regex_solution, sudoku_solution): return ThoughtValidity.VALID_INTERMEDIATE else: return ThoughtValidity.INVALID
######## Testing the MyChecker class above:#######
checker = MyChecker()assert checker.evaluate("", ("3,*,*,2|1,*,3,*|*,1,*,3|4,*,*,1",)) == ThoughtValidity.VALID_INTERMEDIATEassert checker.evaluate("", ("3,4,1,2|1,2,3,4|2,1,4,3|4,3,2,1",)) == ThoughtValidity.VALID_FINALassert checker.evaluate("", ("3,4,1,2|1,2,3,4|2,1,4,3|4,3,*,1",)) == ThoughtValidity.VALID_INTERMEDIATEassert checker.evaluate("", ("3,4,1,2|1,2,3,4|2,1,4,3|4,*,3,1",)) == ThoughtValidity.INVALID
######## Initialize and run the ToT chain, # with maximum number of interactions k set to 30 and # the maximum number child thoughts c set to 8.#######
from langchain_experimental.tot.base import ToTChain
tot_chain = ToTChain(llm=llm, checker=MyChecker(), k=30, c=5, verbose=True, verbose_llm=False)tot_chain.run(problem_description=problem_description)
#######
代理的輸出、迭代和回溯可以在輸出中看到:
> Entering new ToTChain chain...Starting the ToT solve procedure./usr/local/lib/python3.10/dist-packages/langchain/chains/llm.py:278: UserWarning: The predict_and_parse method is deprecated, instead pass an output parser directly to LLMChain. warnings.warn(Thought: 3,4,*,2|1,*,3,*|*,1,*,3|4,*,*,1 Thought: 3,4,1,2|1,*,3,*|*,1,*,3|4,*,*,1 Thought: 3,4,1,2|1,2,3,*|*,1,*,3|4,*,*,1 Thought: 3,4,1,2|1,2,3,4|*,1,*,3|4,*,*,1 Thought: 3,4,1,2|1,2,3,*|1,1,*,3|4,*,*,1 Thought: 3,4,1,2|1,2,3,*|*,2,*,3|4,*,*,1 Thought: 3,4,1,2|1,2,3,*|*,1,1,3|4,*,*,1 Thought: 3,4,1,2|1,2,3,*|*,1,*,4|4,*,*,1 Thought: 3,4,1,2|1,2,3,*|*,1,*,1|4,4,*,1 Thought: 3,4,1,2|1,2,3,*|1,1,*,3|4,*,*,1 Thought: 3,4,1,2|1,2,3,*|*,1,2,3|4,*,*,1 Thought: 3,4,1,2|1,2,3,*|*,1,*,3|4,1,*,1 Thought: 3,4,1,2|1,2,3,*|*,1,*,3|4,*,1,1 Thought: 3,4,1,2|1,*,3,4|*,1,*,3|4,*,*,1 Thought: 3,4,1,2|1,2,3,4|*,1,*,3|4,*,*,1 Thought: 3,4,1,2|1,2,3,4|2,1,*,3|4,*,*,1 Thought: 3,4,1,2|1,2,3,4|2,1,4,3|4,*,*,1 Thought: 3,4,1,2|1,2,3,4|2,1,4,3|4,1,*,* Thought: 3,4,1,2|1,2,3,4|2,1,4,3|4,2,*,* Thought: 3,4,1,2|1,2,3,4|2,1,4,3|4,3,*,* Thought: 3,4,1,2|1,2,3,4|2,1,4,3|4,3,1,* Thought: 3,4,1,2|1,2,3,4|2,1,4,3|4,3,2,* Thought: 3,4,1,2|1,2,3,4|2,1,4,3|4,3,2,1
> Finished chain.3,4,1,2|1,2,3,4|2,1,4,3|4,3,2,1
在Colab筆記本中查看的輸出如下所示:
參考文獻(xiàn):
[1] https://cobusgreyling.medium.com/langsmith-1dd01049c3fb
[2] https://arxiv.org/pdf/2305.08291.pdf
[3]?https://cobusgreyling.medium.com/langchain-langsmith-llm-guided-tree-of-thought-47a2cd5bcfca
文章轉(zhuǎn)自微信公眾號(hào)@ArronAI
對(duì)比大模型API的內(nèi)容創(chuàng)意新穎性、情感共鳴力、商業(yè)轉(zhuǎn)化潛力
一鍵對(duì)比試用API 限時(shí)免費(fèi)