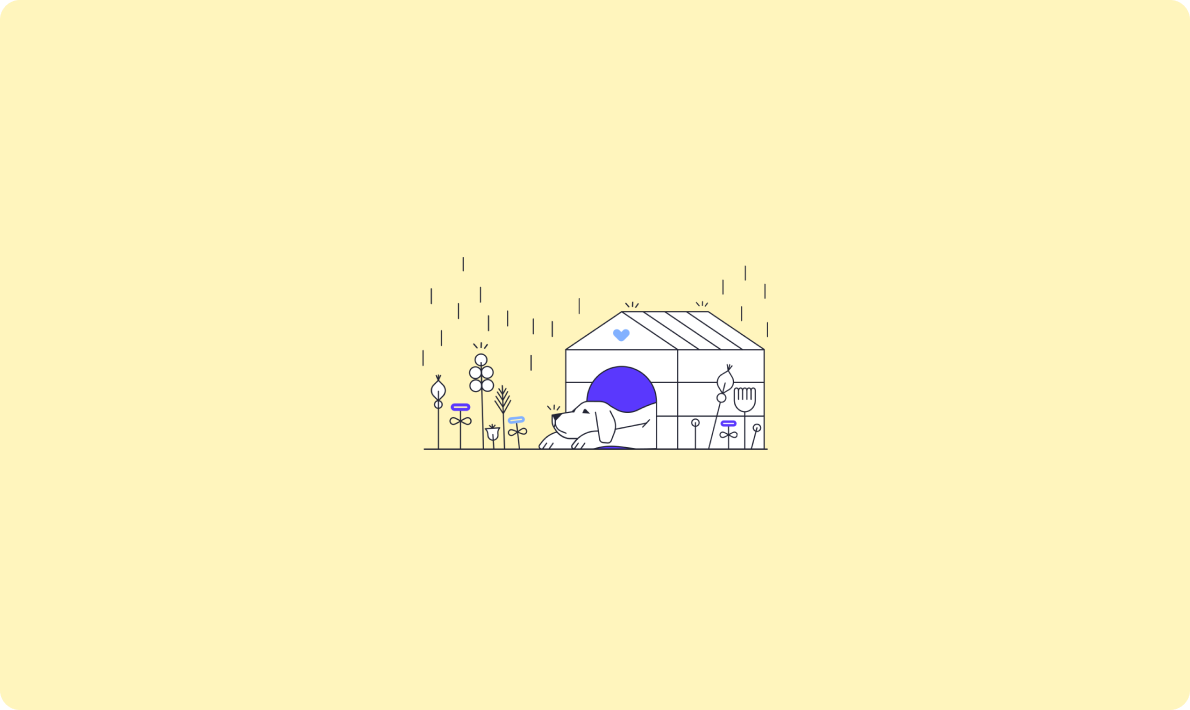
IP歸歸屬地查詢技術(shù)詳解與應(yīng)用
Optuna使用TPE(Tree-structured Parzen Estimator)算法進行貝葉斯優(yōu)化,能夠更智能地選擇下一組實驗參數(shù),從而加速超參數(shù)搜索。
Optuna的設(shè)計簡單而靈活,易于集成到現(xiàn)有的機器學(xué)習(xí)項目中。
提供結(jié)果可視化工具,幫助用戶直觀地了解實驗過程和結(jié)果。
Optuna支持并行優(yōu)化,能夠充分利用計算資源,提高搜索效率。
對于超參數(shù)空間較小或者問題較簡單的情況,Optuna的優(yōu)勢可能不如其他方法顯著。
安裝Optuna非常簡單,可以通過pip安裝:
pip install optuna
或者使用conda安裝:
conda install -c conda-forge optuna
在使用Optuna進行調(diào)參之前,我們需要定義超參數(shù)的搜索空間。
目標函數(shù)是Optuna優(yōu)化超參數(shù)選擇的核心。
使用Optuna的create_study
和optimize
函數(shù)運行優(yōu)化過程。
通過Optuna提供的API獲取找到的最佳超參數(shù)組合。
import optuna
from sklearn import datasets
from sklearn.model_selection import train_test_split
from sklearn.svm import SVC
data = datasets.load_iris()
X_train, X_test, y_train, y_test = train_test_split(data.data, data.target, test_size=0.2)
def objective(trial):
C = trial.suggest_loguniform('C', 1e-5, 1e5)
gamma = trial.suggest_loguniform('gamma', 1e-5, 1e5)
model = SVC(C=C, gamma=gamma)
model.fit(X_train, y_train)
accuracy = model.score(X_test, y_test)
return accuracy
study = optuna.create_study(direction='maximize')
study.optimize(objective, n_trials=100)
best_params = study.best_params
print("最佳超參數(shù):", best_params)
def objective(trial):
params = {
'objective': 'multiclass',
'metric': 'multi_logloss', # Use 'multi_logloss' for evaluation
'boosting_type': 'gbdt',
'num_class': 3, # Replace with the actual number of classes
'num_leaves': trial.suggest_int('num_leaves', 2, 256),
'learning_rate': trial.suggest_loguniform('learning_rate', 0.001, 0.1),
'feature_fraction': trial.suggest_uniform('feature_fraction', 0.1, 1.0),
'bagging_fraction': trial.suggest_uniform('bagging_fraction', 0.1, 1.0),
'bagging_freq': trial.suggest_int('bagging_freq', 1, 10),
'min_child_samples': trial.suggest_int('min_child_samples', 5, 100),
}
model = lgb.LGBMClassifier(**params)
model.fit(X_train, y_train)
y_pred = model.predict_proba(X_val)
loss = log_loss(y_val, y_pred)
return loss
study = optuna.create_study(direction='minimize')
study.optimize(objective, n_trials=50,show_progress_bar=True)
best_params = study.best_params
print(f"Best Params: {best_params}")
def objective(trial):
params = {
'objective': 'multi:softprob', # 'multi:softprob' for multiclass classification
'num_class': 3, # Replace with the actual number of classes
'booster': 'gbtree',
'eval_metric': 'mlogloss', # 'mlogloss' for evaluation
'max_depth': trial.suggest_int('max_depth', 2, 10),
'learning_rate': trial.suggest_loguniform('learning_rate', 0.001, 0.1),
'subsample': trial.suggest_uniform('subsample', 0.1, 1.0),
'colsample_bytree': trial.suggest_uniform('colsample_bytree', 0.1, 1.0),
'min_child_weight': trial.suggest_int('min_child_weight', 1, 10),
}
model = XGBClassifier(**params)
model.fit(X_train, y_train)
y_pred = model.predict_proba(X_val)
loss = log_loss(y_val, y_pred)
return loss
study = optuna.create_study(direction='minimize')
study.optimize(objective, n_trials=50, show_progress_bar=True)
best_params = study.best_params
print(f"Best Params: {best_params}")
確定哪些參數(shù)對模型的整體性能有最顯著的影響。
optuna.visualization.plot_param_importances(study)
模型在多次迭代中的性能。
optuna.visualization.plot_optimization_history(study)
不同超參數(shù)在多次試驗中的進展情況。
optuna.visualization.plot_slice(study, params=['depth', 'learning_rate', 'bootstrap_type'])
optuna.visualization.plot_parallel_coordinate(study)
Optuna作為一個高效的超參數(shù)優(yōu)化工具,在調(diào)參過程中具有明顯的優(yōu)勢。通過智能的搜索策略和輕量級的設(shè)計,它可以顯著減少調(diào)參的時間和計算資源成本。當(dāng)面對大規(guī)模超參數(shù)搜索問題時,Optuna是一個值得考慮的利器,能夠幫助機器學(xué)習(xí)和數(shù)據(jù)科學(xué)領(lǐng)域的從業(yè)者更高效地優(yōu)化模型性能。
問:Optuna支持哪些機器學(xué)習(xí)框架?
答:Optuna支持多種機器學(xué)習(xí)框架,包括Scikit-learn、PyTorch和TensorFlow等。
問:如何定義Optuna的超參數(shù)搜索空間?
答:可以使用Optuna的API定義超參數(shù)的搜索范圍,例如學(xué)習(xí)率、層數(shù)等。
問:如何獲取Optuna找到的最佳超參數(shù)組合?
答:通過Optuna提供的API可以獲取找到的最佳超參數(shù)組合,例如study.best_params
。
問:Optuna的可視化功能有哪些?
答:Optuna提供了多種可視化工具,包括參數(shù)重要性圖、優(yōu)化歷史圖、參數(shù)切片圖和平行坐標圖等。
問:Optuna是否支持并行優(yōu)化?
答:是的,Optuna支持并行優(yōu)化,能夠充分利用計算資源,提高搜索效率。