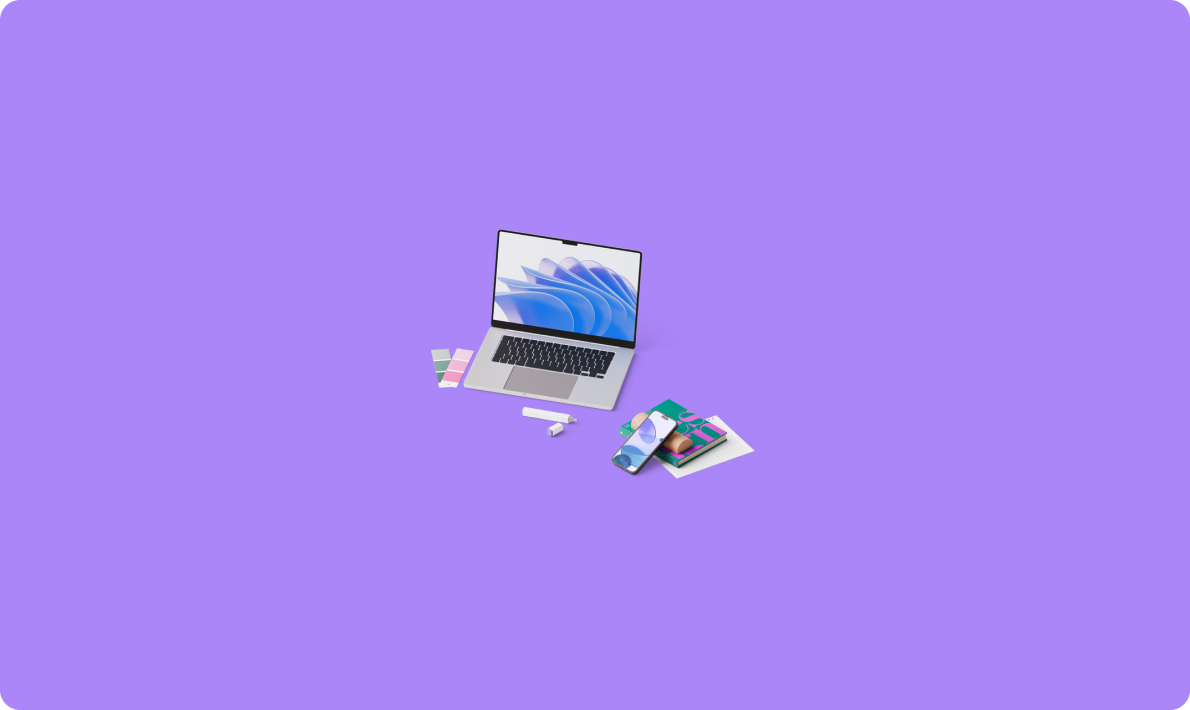
中文命名實體識別(Named Entity Recognition, NER)初探
在Pytorch中,位置編碼的實現可以通過繼承nn.Module類來創建一個自定義的模塊。這個模塊需要在初始化時生成位置編碼矩陣,并在前向傳播時將編碼加到輸入的嵌入向量中。
以下是一個簡單的Pytorch位置編碼實現示例:
class PositionalEncoding(nn.Module):
def __init__(self, dim, dropout, max_len=5000):
super(PositionalEncoding, self).__init__()
if dim % 2 != 0:
raise ValueError("Cannot use sin/cos positional encoding with odd dim (got dim={:d})".format(dim))
pe = torch.zeros(max_len, dim) # max_len 是解碼器生成句子的最長的長度,假設是 10
position = torch.arange(0, max_len).unsqueeze(1)
div_term = torch.exp((torch.arange(0, dim, 2, dtype=torch.float) * -(math.log(10000.0) / dim)))
pe[:, 0::2] = torch.sin(position.float() * div_term)
pe[:, 1::2] = torch.cos(position.float() * div_term)
pe = pe.unsqueeze(1)
self.register_buffer('pe', pe)
self.drop_out = nn.Dropout(p=dropout)
self.dim = dim
def forward(self, emb, step=None):
emb = emb * math.sqrt(self.dim)
if step is None:
emb = emb + self.pe[:emb.size(0)]
else:
emb = emb + self.pe[step]
emb = self.drop_out(emb)
return emb
這個代碼片段展示了如何在Pytorch中實現位置編碼,其中包括初始化編碼矩陣,定義前向傳播過程,并使用sin和cos函數生成編碼。
在VisionTransformer中,位置編碼被設計為可學習的。這意味著在訓練過程中,模型可以調整位置編碼以優化性能。下面是一個實現可學習絕對位置編碼的代碼示例:
self.pos_embed = nn.Parameter(torch.zeros(1, num_patches + self.num_tokens, embed_dim))
def forward_features(self, x):
# [B, C, H, W] -> [B, num_patches, embed_dim]
x = self.patch_embed(x) # [B, 196, 768]
# [1, 1, 768] -> [B, 1, 768]
cls_token = self.cls_token.expand(x.shape[0], -1, -1)
if self.dist_token is None:
x = torch.cat((cls_token, x), dim=1) # [B, 197, 768]
else:
x = torch.cat((cls_token, self.dist_token.expand(x.shape[0], -1, -1), x), dim=1)
x = self.pos_drop(x + self.pos_embed)
相對位置編碼是一種改進的編碼方法,能夠捕捉序列中元素之間的相對位置信息。在SwinTransformer中,相對位置編碼被廣泛應用于圖像處理任務。
以下代碼展示了如何在Pytorch中實現相對位置編碼:
class RelativePositionBias(nn.Module):
def __init__(self, num_heads, h, w): # (4,16,16)
super().__init__()
self.num_heads = num_heads #4
self.h = h #16
self.w = w #16
self.relative_position_bias_table = nn.Parameter(
torch.randn((2 * h - 1) * (2 * w - 1), num_heads) * 0.02) # (961,4)
coords_h = torch.arange(self.h) # [0,16]
coords_w = torch.arange(self.w) # [0,16]
coords = torch.stack(torch.meshgrid([coords_h, coords_w])) # (2, 16, 16)
coords_flatten = torch.flatten(coords, 1) # (2, 256)
relative_coords = coords_flatten[:, :, None] - coords_flatten[:, None, :] #(2,256,256)
relative_coords = relative_coords.permute(1, 2, 0).contiguous() #(256,256,2)
relative_coords[:, :, 0] += self.h - 1 #(256,256,2)
relative_coords[:, :, 1] += self.w - 1
relative_coords[:, :, 0] *= 2 * self.h - 1
relative_position_index = relative_coords.sum(-1) # (256, 256)
self.register_buffer("relative_position_index", relative_position_index)
def forward(self, H, W):
relative_position_bias = self.relative_position_bias_table[self.relative_position_index.view(-1)].view(self.h,self.w,self.h * self.w,-1) # h, w, hw, nH (16,16,256,4)
relative_position_bias_expand_h = torch.repeat_interleave(relative_position_bias, H // self.h,dim=0) # (在dim=0維度重復7次)->(112,16,256,4)
relative_position_bias_expanded = torch.repeat_interleave(relative_position_bias_expand_h, W // self.w,dim=1) # HW, hw, nH #(在dim=1維度重復7次)
relative_position_bias_expanded = relative_position_bias_expanded.view(H * W, self.h * self.w,
self.num_heads).permute(2, 0,1).contiguous().unsqueeze(0)
return relative_position_bias_expanded
相對位置編碼在捕捉序列的相對位置信息方面具有顯著優勢,尤其是在處理長序列或大尺寸圖像時。相較于絕對位置編碼,相對位置編碼可以更好地泛化到不同的輸入尺寸和不同的任務場景。
盡管相對位置編碼帶來了許多性能上的提升,但其實現也面臨一些挑戰。主要問題在于計算復雜度的增加,以及如何有效地在不同任務中調整編碼參數。
位置編碼在許多自然語言處理和計算機視覺任務中都有廣泛應用。具體來說,在機器翻譯、文本摘要、語義分割等任務中,位置編碼都發揮了重要作用。
通過本文的討論,我們了解到位置編碼在Transformer模型中的重要性,以及Pytorch中實現位置編碼的幾種方法。位置編碼不僅增強了模型捕捉序列順序信息的能力,還為復雜任務提供了更好的泛化性能。
問:位置編碼在Transformer中有什么作用?
問:Pytorch中如何實現位置編碼?
問:相對位置編碼有哪些優勢?
問:位置編碼在計算機視覺任務中的應用是什么?