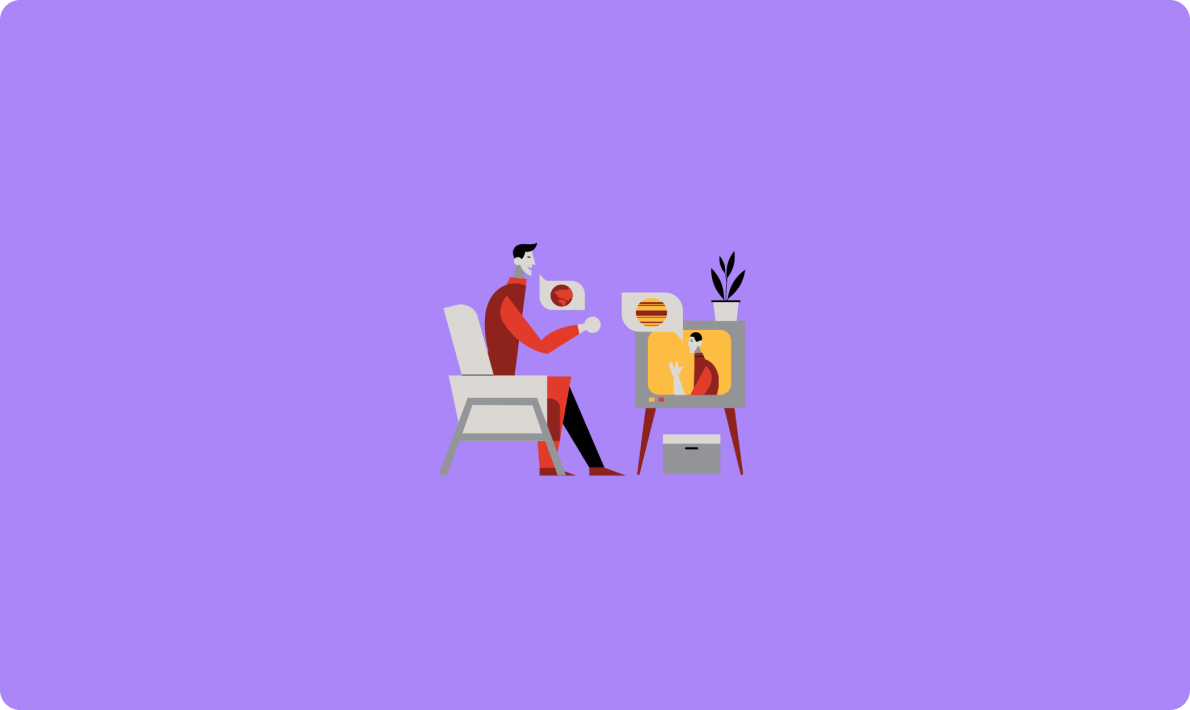
有道翻譯API:開(kāi)啟多語(yǔ)言交流新篇章
下一步是從嵌入向量列表創(chuàng)建 FAISS 索引。FAISS 為相似性搜索提供了各種距離度量,包括內(nèi)積 (IP) 和 L2(歐幾里得)距離。
FAISS 還提供各種索引選項(xiàng)。它可以使用近似或壓縮技術(shù)來(lái)有效處理大型數(shù)據(jù)集,同時(shí)平衡搜索速度和準(zhǔn)確性。在本教程中,我們將使用“平面”索引,它通過(guò)將查詢向量與數(shù)據(jù)集中的每個(gè)向量進(jìn)行比較來(lái)執(zhí)行強(qiáng)力搜索,以確保以更高的計(jì)算復(fù)雜度為代價(jià)獲得準(zhǔn)確的結(jié)果。
def create_faiss_index(embeddings, image_paths, output_path):
dimension = len(embeddings[0])
index = faiss.IndexFlatIP(dimension)
index = faiss.IndexIDMap(index)
vectors = np.array(embeddings).astype(np.float32)
# Add vectors to the index with IDs
index.add_with_ids(vectors, np.array(range(len(embeddings))))
# Save the index
faiss.write_index(index, output_path)
print(f"Index created and saved to {output_path}")
# Save image paths
with open(output_path + '.paths', 'w') as f:
for img_path in image_paths:
f.write(img_path + '\n')
return index
OUTPUT_INDEX_PATH = "/content/vector.index"
index = create_faiss_index(embeddings, image_paths, OUTPUT_INDEX_PATH)
faiss.IndexFlatIP
初始化內(nèi)積相似度索引,將其包裝在 faiss.IndexIDMap
中,以將每個(gè)向量與 ID 關(guān)聯(lián)。接下來(lái), index.add_with_ids
將向量添加到具有連續(xù) ID 的索引中,并將索引與圖像路徑一起保存到磁盤。
索引可以立即使用,也可以保存到磁盤以備將來(lái)使用。要加載 FAISS 索引,我們將使用以下函數(shù):
def load_faiss_index(index_path):
index = faiss.read_index(index_path)
with open(index_path + '.paths', 'r') as f:
image_paths = [line.strip() for line in f]
print(f"Index loaded from {index_path}")
return index, image_paths
index, image_paths = load_faiss_index(OUTPUT_INDEX_PATH)
建立 FAISS 索引后,我們現(xiàn)在可以使用文本查詢或參考圖像檢索圖像。如果查詢是圖像路徑,則使用 PIL的 Image.open
打開(kāi)查詢。接下來(lái),使用 CLIP的 model.encode
提取查詢嵌入向量。
def retrieve_similar_images(query, model, index, image_paths, top_k=3):
# query preprocess:
if query.endswith(('.png', '.jpg', '.jpeg', '.tiff', '.bmp', '.gif')):
query = Image.open(query)
query_features = model.encode(query)
query_features = query_features.astype(np.float32).reshape(1, -1)
distances, indices = index.search(query_features, top_k)
retrieved_images = [image_paths[int(idx)] for idx in indices[0]]
return query, retrieved_images
檢索發(fā)生在 index.search
方法上。它實(shí)現(xiàn)了 k-最近鄰 (kNN) 搜索,以找到與查詢向量最相似的 k 個(gè)向量。我們可以通過(guò)更改 top_k
參數(shù)來(lái)調(diào)整 k 的值。在我們的實(shí)現(xiàn)中,kNN 搜索中使用的距離度量是余弦相似度。該函數(shù)返回查詢和檢索圖像路徑的列表。
現(xiàn)在我們準(zhǔn)備使用文本查詢進(jìn)行搜索。輔助函數(shù) visualize_results
顯示結(jié)果。你可以在關(guān)聯(lián)的 Colab 筆記本中找到它。讓我們探索針對(duì)文本查詢“ball”檢索到的最相似的 3 幅圖像,例如:
query = 'ball'
query, retrieved_images = retrieve_similar_images(query, model, index, image_paths, top_k=3)
visualize_results(query, retrieved_images)
對(duì)于查詢“animal”,我們得到:
使用參考圖像搜索:
query ='/content/drive/MyDrive/Colab Notebooks/my_medium_projects/Image_similarity_search/image_dataset/pexels-w-w-299285-889839.jpg'
query, retrieved_images = retrieve_similar_images(query, model, index, image_paths, top_k=3)
visualize_results(query, retrieved_images)
如我們所見(jiàn),我們?yōu)楝F(xiàn)成的預(yù)訓(xùn)練模型獲得了非常酷的結(jié)果。當(dāng)我們通過(guò)眼睛繪畫的參考圖像進(jìn)行搜索時(shí),除了找到原始圖像外,它還找到了一個(gè)匹配的眼鏡和另一幅畫。這展示了查詢圖像語(yǔ)義含義的不同方面。
在本教程中,我們使用 CLIP 和 FAISS 構(gòu)建了一個(gè)基本的圖像相似性搜索引擎。檢索到的圖像與查詢具有相似的語(yǔ)義含義,表明該方法的有效性。
雖然 CLIP 對(duì)于零樣本模型顯示出不錯(cuò)的結(jié)果,但它在分布外數(shù)據(jù)、細(xì)粒度任務(wù)上可能表現(xiàn)出較低的性能,并繼承了訓(xùn)練數(shù)據(jù)的自然偏差。為了克服這些限制,你可以嘗試其他類似 CLIP 的預(yù)訓(xùn)練模型(如 OpenClip),或者在自己的自定義數(shù)據(jù)集上微調(diào) CLIP。
原文鏈接:http://www.bimant.com/blog/image-search-engine-diy/
有道翻譯API:開(kāi)啟多語(yǔ)言交流新篇章
如何免費(fèi)調(diào)用手機(jī)歸屬地查詢API實(shí)現(xiàn)用戶定位
WordPress REST API 初學(xué)者指南
Python 輕松實(shí)現(xiàn)天氣提醒:通過(guò)天氣實(shí)況 API 獲取城市氣溫
將 API 貨幣化:加速增長(zhǎng)并減輕工程師的壓力
API治理:有效API管理的最佳實(shí)踐和策略
API優(yōu)先設(shè)計(jì):構(gòu)建可擴(kuò)展且靈活的軟件的現(xiàn)代方法
API 安全:如何準(zhǔn)備和應(yīng)對(duì)攻擊漏洞
Python 集成 NFT構(gòu)建 API 的實(shí)戰(zhàn)指南
對(duì)比大模型API的內(nèi)容創(chuàng)意新穎性、情感共鳴力、商業(yè)轉(zhuǎn)化潛力
一鍵對(duì)比試用API 限時(shí)免費(fèi)