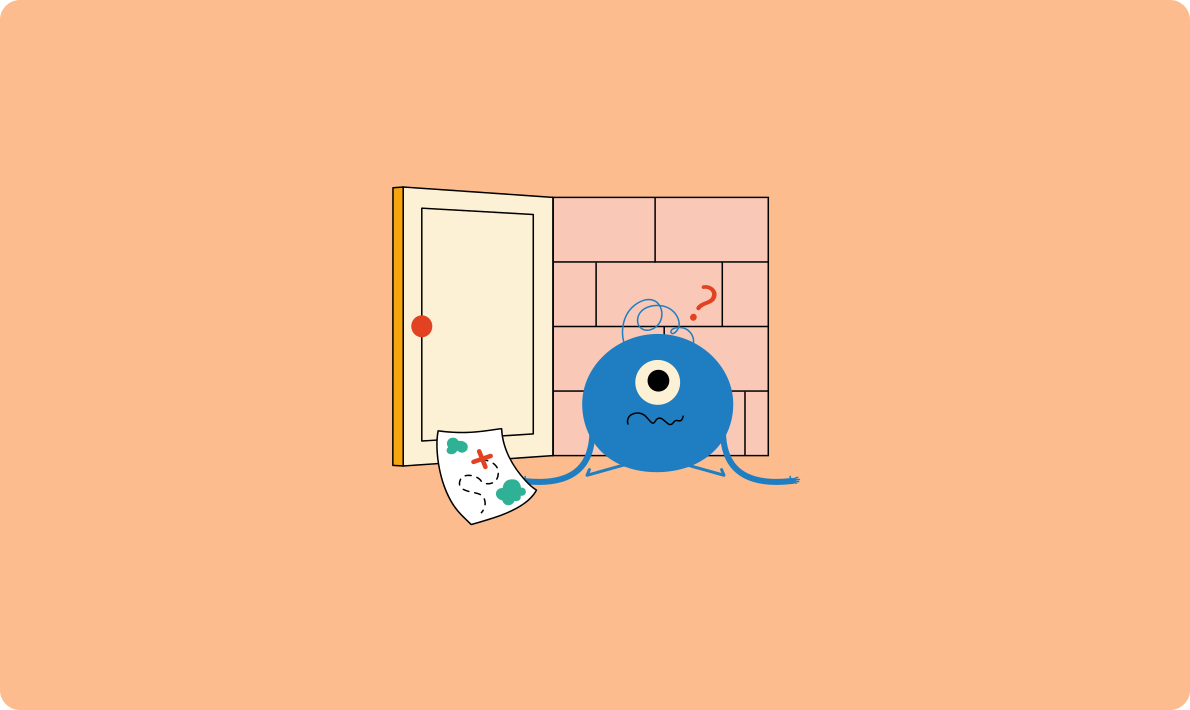
掌握API網關認證:安全連接的可靠方法
python
深色版本
1import speech_recognition as sr
2
3def transcribe_audio(audio_file):
4 recognizer = sr.Recognizer()
5
6 with sr.AudioFile(audio_file) as source:
7 audio_data = recognizer.record(source)
8 text = recognizer.recognize_google(audio_data, language='zh-CN')
9
10 return text
創(chuàng)建一個違規(guī)詞庫,這些詞庫可以包括敏感詞匯、禁止使用的語言等。
python
深色版本
1def load_word_list(file_path):
2 with open(file_path, 'r', encoding='utf-8') as file:
3 words = file.readlines()
4 return [word.strip() for word in words]
創(chuàng)建一個文件(例如 forbidden_words.txt
),并在其中列出所有需要攔截的詞匯。
編寫一個函數來檢查文本是否包含違規(guī)詞匯。
python
深色版本
1def check_forbidden_words(text, forbidden_words):
2 for word in forbidden_words:
3 if word in text:
4 return True
5 return False
現在我們可以整合這些步驟來創(chuàng)建一個完整的違規(guī)詞檢測系統(tǒng)。
python
深色版本
1def main():
2 # 加載違規(guī)詞庫
3 forbidden_words = load_word_list('forbidden_words.txt')
4
5 # 錄音文件路徑
6 audio_file = 'example.wav'
7
8 # 轉換音頻為文本
9 text = transcribe_audio(audio_file)
10
11 # 檢查違規(guī)詞
12 if check_forbidden_words(text, forbidden_words):
13 print("違規(guī)詞檢測到!")
14 print(f"違規(guī)內容: {text}")
15 else:
16 print("未檢測到違規(guī)詞。")
17
18if __name__ == "__main__":
19 main()
為了更全面地檢測違規(guī)內容,您可以考慮加入以下功能:
部署您的應用并設置適當的監(jiān)控和日志記錄機制,以便于跟蹤系統(tǒng)性能和故障排查。
這個教程提供了一個基礎框架,您可以根據具體需求對其進行擴展和優(yōu)化。例如,您可能需要增加機器學習模型來提高違規(guī)詞識別的準確率,或者使用云服務提供商提供的更高級的語音識別和自然語言處理服務。